The Great AI Resource Arm Wrestle: Learning vs Inference
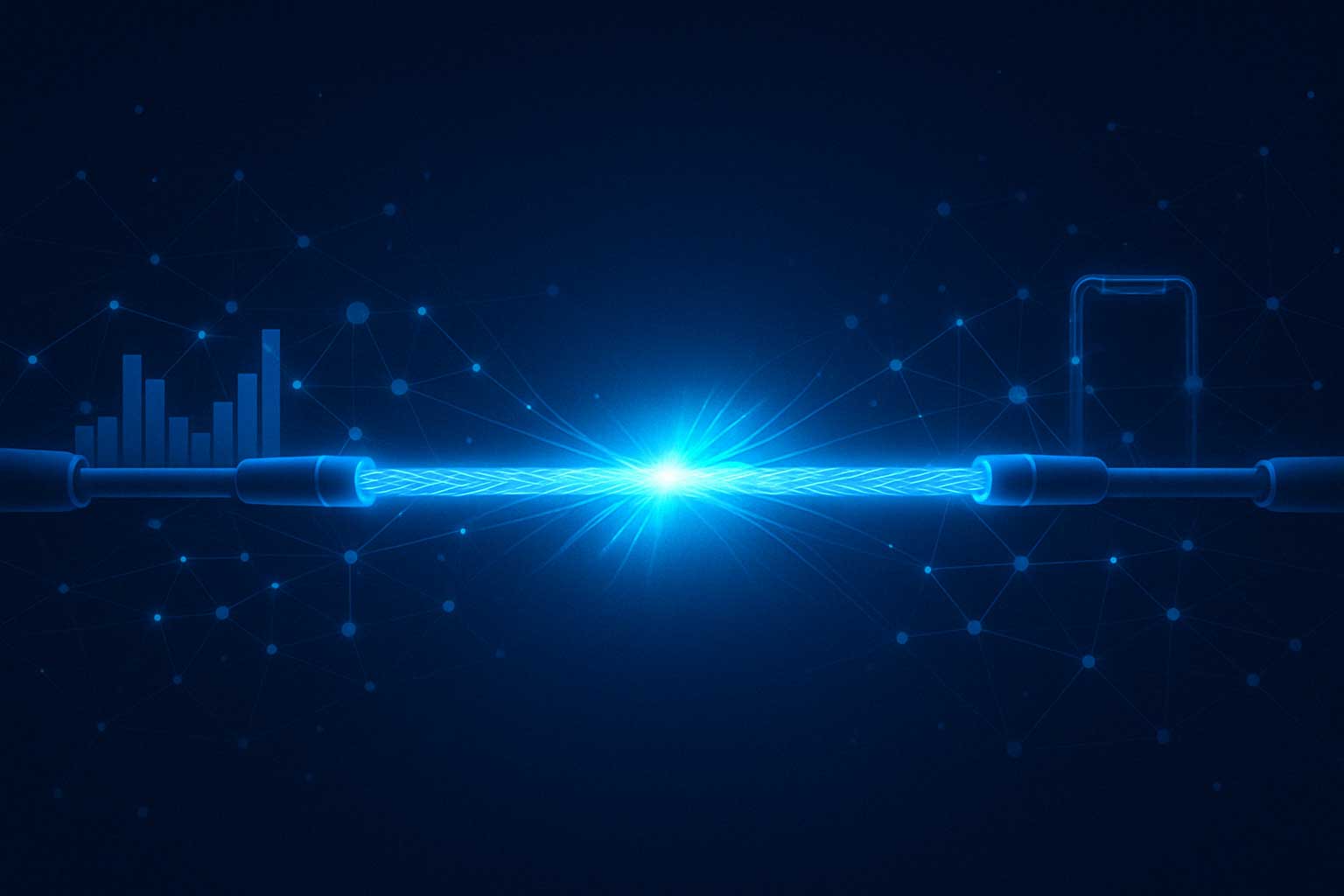
AI is evolving through distinct step changes, with each unlocking new capabilities and opportunities. First, traditional AI and early machine learning models automated structured tasks. Then came Generative AI and LLMs—tools that don’t just respond, they create. From writing and coding to summarising, translating and simulating human conversation, they’ve changed the way we interact with machines. Today, Agentic AI doesn’t just answer questions, it sets goals, figures out how to achieve them, and carries out multi-step tasks on its own.
Now, we stand on the edge of the next major step change: Reasoning AI. With the ability to solve complex problems, these powerful Reasoning AI models are driving a new era of intelligence, and with it a new era of booming data centre demand.
What is Reasoning AI and how is it different to traditional AI models?
Unlike traditional generative AI models, which predict the next word or pixel based on statistical probabilities and existing patterns, Reasoning AI can think through problems before providing an answer. It operates like human reasoning— taking multiple steps to arrive at a conclusion rather than regurgitating learned patterns.
This tectonic shift represents a massive leap forward in what is possible with AI. While traditional chat models, like ChatGPT, can generate fluent responses, they struggle with complex problem-solving, logical reasoning, and multi-step decision-making.
Reasoning AI, on the other hand, processes information in depth, refining its response as it goes. This leads to more intelligent, more accurate, and more thoughtful outputs.
I’m sure most who have used traditional LLMs have experienced the frustration of inaccurate or incomplete answers. While they excel at generating content, their reliability for critical decisions is limited. Reasoning AI changes that. Though it requires more compute per task, it delivers significantly more accurate responses thus eliminating the ‘waste’ of incorrect outputs. At first glance, it may seem more resource-intensive, but in reality, it reduces the need for endless prompting, potentially making it far more efficient in practice.
What are the experts saying?
We in the industry are acknowledging this shift towards Reasoning AI models. Jensen Huang, CEO of NVIDIA, said in a recent earnings call “The more the model thinks, the smarter the answer.” Marking the growing importance of depth and accuracy alongside speed and efficiency for AI models.
NVIDIA launched an entire suite of Reasoning AI tools, the Llama Nemotron reasoning family, which it unveiled at this years GTC Conference in March. Shortly after Microsoft released two new agents for it’s 365 Copilot, Researcher and Analyst, which they claim use “deep reasoning” to “perform complex tasks and make more accurate decisions.” while Google released Gemini 2.5 models, a reasoning model which is “capable of reasoning through thoughts before responding, resulting in enhanced performance and improved accuracy.”
The industry is moving fast. Data centres will need to keep pace and meet this growing demand. NVIDIA’s CFO, Colette Kress explained “Long-thinking, reasoning AI can require 100 times more compute per task compared to one-shot inferences.” This has profound implications for how we design and scale AI infrastructure.
What does this mean for data centres?
One thing is clear: Reasoning AI will require more compute than ever before.
While the training of AI models has always been computationally intense, the inference stage (the use of the model to answer questions or make decisions) has typically been comparatively less intensive. That’s changing. As Reasoning AI develops, inference is expected to become the larger driver of compute demand. This is something we’ve already started to see in recent new entrants to the AI market.
Jensen and others predict that as AI training becomes more efficient, the real long-term challenge will be serving millions, even billions, of Reasoning AI queries in real-time as these tools become more of a part of our everyday life.
For the data centre industry this means increased demand for larger data centres closer to the end users in major cities. This will allow for the lowest latency possible for inferencing.